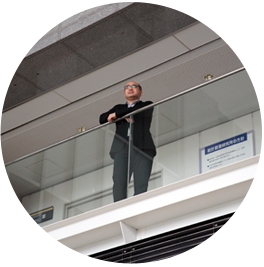
Professor Tomoyuki Higuchi has been leading projects related to Data Assimilation and Simulation Support Technologies at the Research Commons since the inception of the Transdisciplinary Research Integration Center in Inter-University Research Institute Corporation Research Organization of Information and Systems (ROIS). Today, as the Director-General of the Institute of Statistical Mathematics (ISM), one of four institutes in ROIS, he is working on continuous reformation of the only research institute in Japan. ISM is looking into the mathematical principles of probability and their application. The promotion of collaborative research and joint use and professional development such as data scientists, which is core research and educational activities in ISM. These activities are mutual with the Research Commons, which promotes data-centric science. Recently, Professor Higuchi has been expanding “Network of Excellence (NOE)”, a domestic and international research network based at ISM, as a support structure for the cutting-edge research. Professor Higuchi discusses what kinds of systems in research institutions are the driving factors in future science projects for which "data-centric" is becoming an increasingly important keyword.
Teamwork in support of cutting-edge research
In this "Big Data Era", we cannot engage in cutting-edge research without teamwork. An individual conducting cutting-edge research on his or her own is almost unheard of these days. Under these circumstances, team-building becomes a very important driving force for advanced research. It requires individuals to take on different roles such as organizing and planning, and it is also important for making diverse career paths available. In the U.S., for example, there is a job title called "scientific programmer," in which individuals are hired for research projects using external funds to make professional and advanced contributions. This demonstrates that research can be advanced tremendously by improving its method of employment.
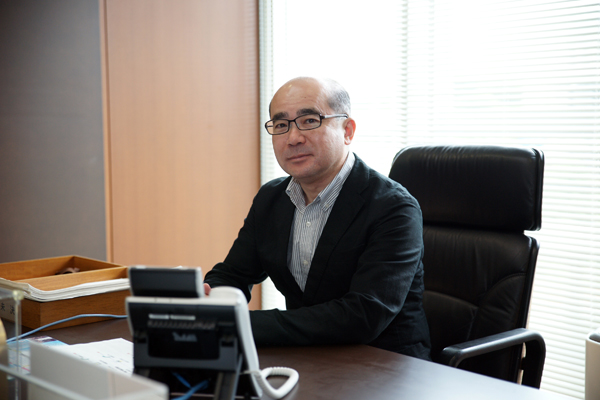
From individual developments to team-oriented professional developments
Looking back on Transdisciplinary Research Integration Center during the first medium term plan of ROIS, which preceded the Research Commons now, I remember that the existing four research institutes belonged to ROIS got together for the first time, and we started to build a system from scratch. While it was difficult at the beginning, the teamwork involved gave it a hand-made feel. We did not know how valuable our research abilities were, but we collaborated in order to give it a try together, because we were interested in integrating different fields. In Research Commons, (which has inherited of the concept of integrated research), projects and management have run much more smoothly, although some aspects have room for improvement. Specifically, from the next term onward, we need to consciously build a system that we can develop integrated professionals, instead of searching for successors on the outside.
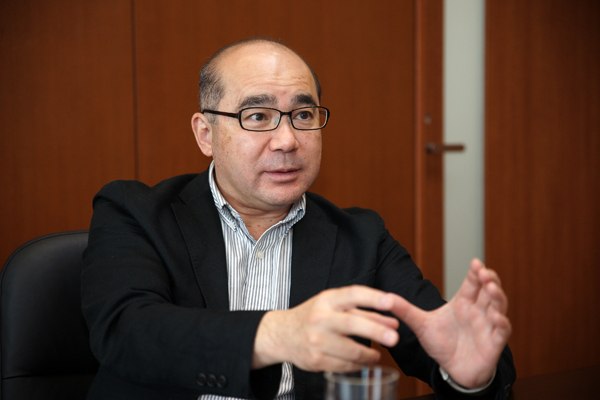
Making the institutes' roles clearer and more advanced
Unlike 10 years ago when ROIS was established, the frictions between our country and the neighboring countries keep increasing. In relation to various phenomena and problems in this complex modern society, we make statistical predictions and mathematical analyze risks including related matters. We also come up with methods for controlling policies and ways to improve optimization in order to take advantage of our limited resources. In order to fulfill the role, we set three goals for our institute. The first goal is to improve the functionality of open-type research collaboration. We aim to create teams such that they choose more challenging and interesting topics whose outcomes cannot be predicted. The second goal is to reinforce the "Project for Fostering and Promoting of Statistical Thinking" for developing professionals who can approach projects from a statistical perspective. The third goal is to network statistical mathematics on a global scale. For example, our institute has signed many MOUs (Memorandum of Understanding) with international institutions.
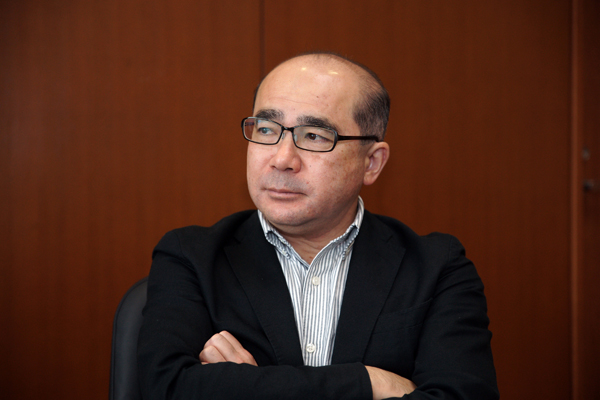
Predict, analyze, control, and optimize constantly changing targets
Today, statistics uses data manipulation as a base to help people make reasonable decisions, for example, regarding the effectiveness and safety standards in medicine, risk management in finance, and quality control in factories. Statistics, however, is much more than such examples. For me, one of the challenges of “Data Assimilation” is to connect the simulations as forward inference and data analysis as backward inference. The other challenge is to predict, to analyze, and to control not static and fixed phenomena but the continuously changing dynamic phenomena as targets. Uncertainty cannot be avoided in the complex phenomena of modern science. In my view of statistics, there are two types of machine learning. One is artificial intelligence (AI), which includes Bayesian inference and deep-learning that aims to emulate the human brain's ability to process information; the other type is based on mathematics to the utmost limit, which has the possibility to produce insights that cannot be made from the large scale data of the past. Our goal is to work on both in "Data Assimilation and Simulation Support Technologies."
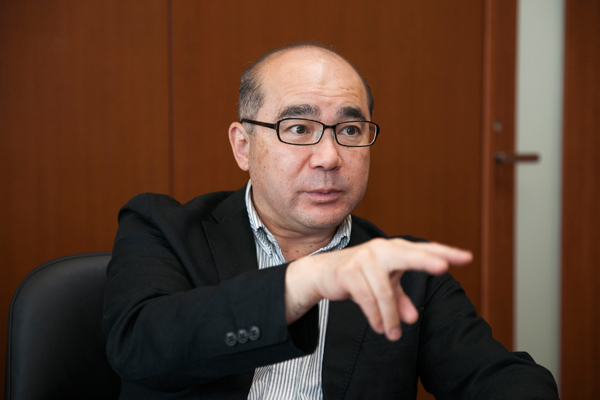
The key to using data-centric science for innovations
The other day, I had the chance to talk to Professor Guo, the director of the Data Science Institute at the Imperial College in London, and he told me that four keywords characterize data-centric science: Intelligence, Integration, Interdisciplinary, and Interaction. What struck me then was that these were the "4Is (four “I”s mentioned above) for I (Innovation)" in these present days. Intelligence is at the forefront, but breakthroughs come about only when strong interdisciplinary efforts are integrated. I think the last keyword, Interaction, is very important; it could refer to interactions between humans and humans or between humans and machines. We aim to integrate what we have learned over the past ten years into our structure and then develop it. Now is the time when we use data-centric science and start to produce results related to society more strongly.
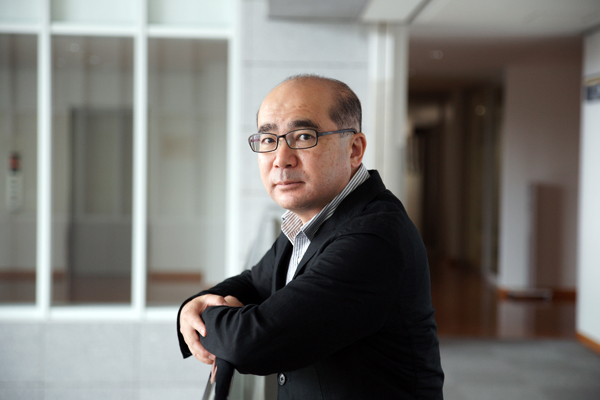
(Text in Japanese: Tomoyuki Higuchi, Rue Ikeya. Photographs: Mitsuru Mizutani. Published: July 10, 2015)