Here, we introduce the Data Assimilation and Simulation Support Technologies project, one of the three foundational projects of the Data-Centric Science Research Commons. This project deals with modeling and analysis of data. Data assimilation can be described as a statistical method for connecting simulation and data analysis. Important aspect of data assimilation is its wide scope of applicability, from physical and biological systems through to economic systems such as marketing; it can assist in almost any field. Through the project, young participants learn about statistical science and become familiar with handling large data and programming. Thus, the project is ta good preparation ground for the human resources that will be needed in the era of big data. Project leader, Junji Nakano (The Institute of Statistical Mathematics) introduces the project.
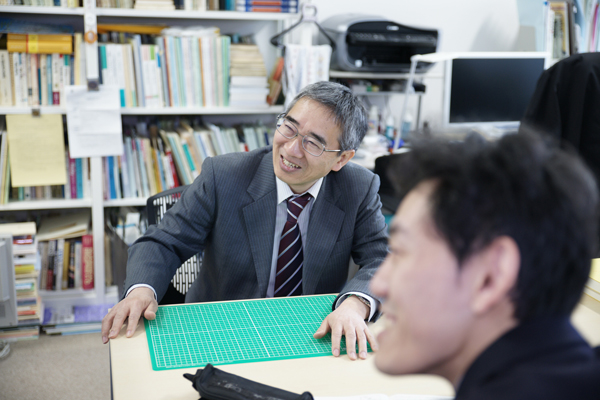
Hidden Background Structure behind Observed Phenomena
This project was originally started by the Institute of Statistical Mathematics’ Director General Tomoyuki Higuchi, and we have continued it, working in collaboration with groups from the National Institute of Polar Research and the National Institute of Genetics, together with the Research and Development Center for Data Assimilation of the Institute of Statistical Mathematics. Statistics does not focus only on therory: it uses data to understand the hidden structure in the background behind stochastic phenomena. Statistician and geneticist Sir Ronald Aylmer Fisher (1890-1962) established the statistical theory that have continued to the present because he considered how to find hidden background mechanisms from small experimental data sets of 10 or 20 items. The ability to think about data that has not yet been obtained on the basis of present data and to make predictions about it, comes from the power of modeling.
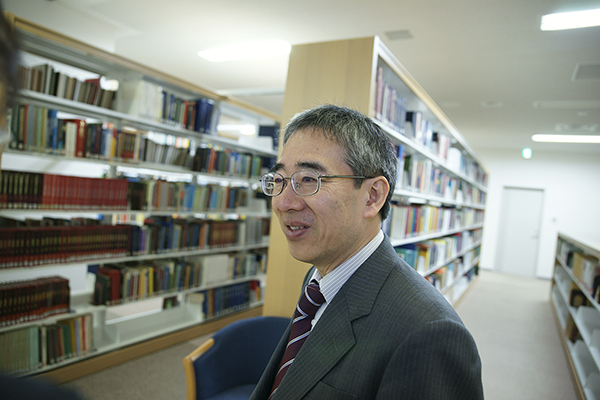
Two types of Simulations
In fields where mathematical equations can describe phenomena sufficiently, simulations and modeling have been used for a long time. Often a physical simulation is conducted, with which a definite single model is decided upon, and then appropriate initial values are fed into it to see a future behavior. By comparison, in a statistical simulation, the factor of uncertainty plays a major role. When there is a large volume of data that can be explained by unspecified formula, we must estimate which is the best to use. For this task, we assign certain values and calculate the discrepancy between the model and the actual data. Then we select the model with the least error for the data, and investigate again in the area around its parameters. This operation is performed repeatedly. Random numbers are effective for generating such kind of data and a Bayesian technique called a “particle filter” is used to find a model that fits the data well.
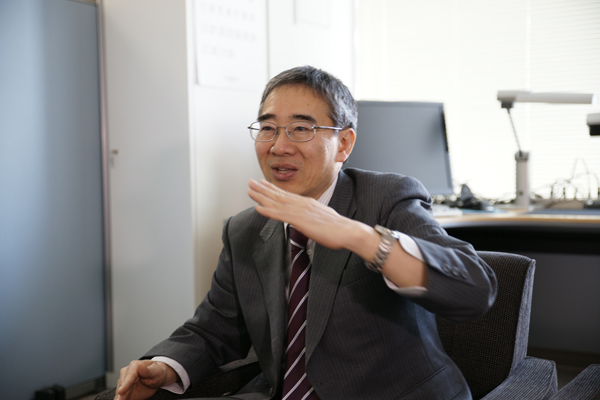
What is Data Assimilation?
Simulation models generated by repeated calculations based on random numbers may be problematic when compared to actual data. Here, we could progressively improve the accuracy by using actual data and fitting the model so that its results match the actual data, making it closer to the real world. This process is what we call data assimilation. Among the phenomena in human societies, there are some things that we cannot control at any cost. Quantum mechanics has indicated that uncertainty is an inherent characteristic even in physical phenomena. Errors are unavoidable, and so is incompleteness of data. Our approach for handling such events is to use statistics and data assimilation. In other words, it is more realistic to use these tools.
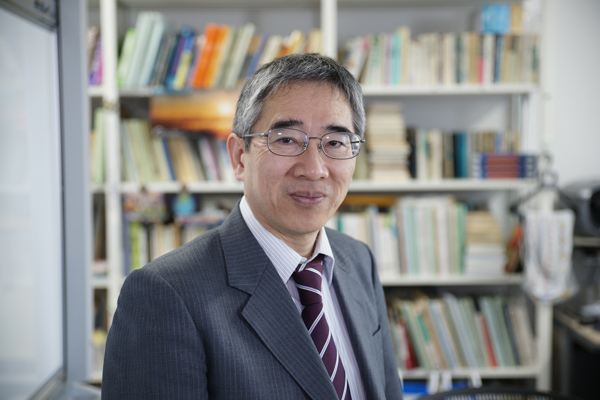
Collaborative research to Find a Useful Method
Our project includes three teams: Mathematical theory and Computing, Modeling, and Data Design. Each team is led by the Institute of Statistical Mathematics, the National Institute of Polar Research, and the National Institute of Genetics, respectively. As statistics is a discipline that has built its methods in accordance with objectives based on what people need to know while creating and examining data, there is plenty of exchanges among the institutes. For example, statistics can propose answers for the possibility of classifying a certain difference with a certain experiment, or for decisions regarding which kind of experiment is needed to express target information with as few experiments as possible. The Data Design team is working on this kind of joint research with members from the National Institute of Genetics and the Institute of Statistical Mathematics. Statisticians strive to create methods that are as versatile as possible, because such methods can not only be effectively used in a wide range of fields, but also will be used for many years to come.
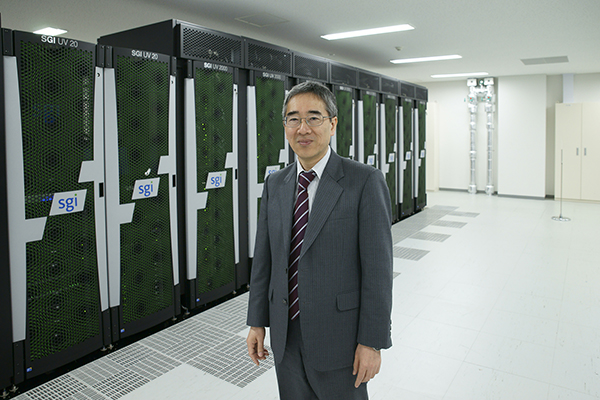
(Text in Japanese: Junji Nakano, Rue Ikeya. Photographs: Mitsuru Mizutani. Published: May 12, 2014)