What’s on the Horizon in the Changing World of Big Data?
We are living in the era of big data. Easy access to colossal amounts of data being constantly gathered and compiled on virtually every topic imaginable is enabling businesses and research institutions, such as universities, to gain insights that would have seemed impossibly far-fetched only a few years ago. As artificial intelligence (AI) becomes more sophisticated in its ability to autonomously make data-driven decisions, many of us are asking ourselves how soon we may reach the singularity — the tipping point where computer intelligence exceeds that of humans. This causes us to pause and examine in which directions data science is advancing and what kind of research we need to conduct to help solve complex problems. The directors of the National Institute of Informatics (NII) and the Institute of Statistical Mathematics (ISM) – which are both part of the Research Organization of Information and Systems (ROIS) – recently sat down to talk about all these issues and share their outlooks on the future of big data with the Science Report’s readers. Here are their perspectives and the latest on the leading-edge technology and research.
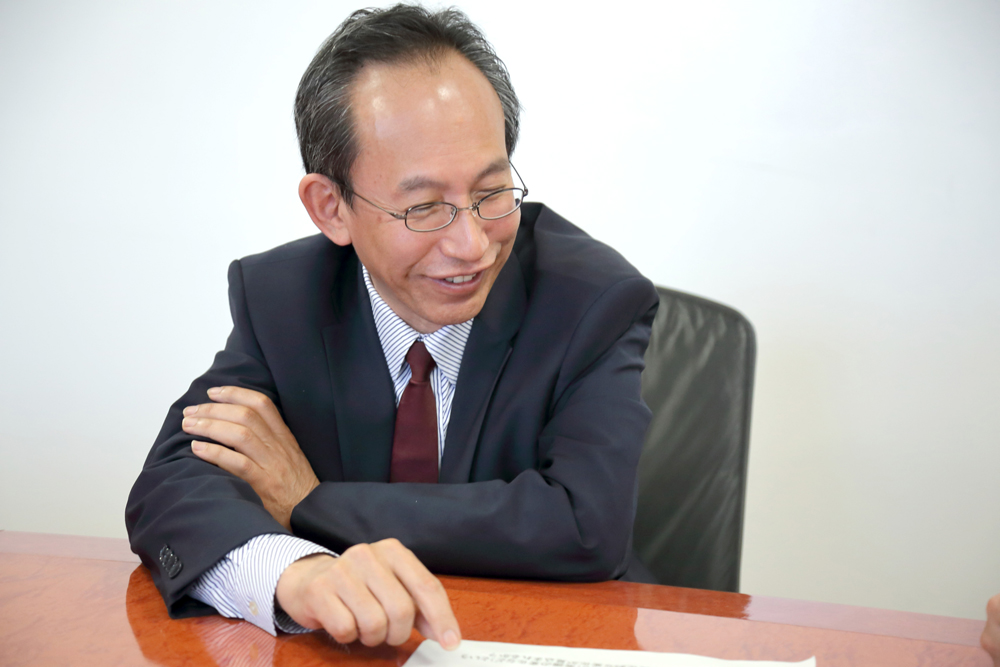
Ask the Experts: Masaru Kitsuregawa (National Institute of Informatics)
Since 2013, Dr. Kitsuregawa has served as the director of the National Institute of Informatics, the Research Organization of Information and Systems, and professor at the Institute of Industrial Science at the University of Tokyo. As an expert in database engineering, he leads some of the most prominent informatics research projects in Japan and has developed a high-speed database engine, using “out-of-order execution schemes.” He is known for operating the mammoth Global Environmental Database. The 1983 graduate of the University of Tokyo holds a Ph.D. in engineering from the same university. He previously served as vice chairman of the Information Processing Society of Japan and 23rd chairman of the Informatics Committee of the Science Council of Japan.
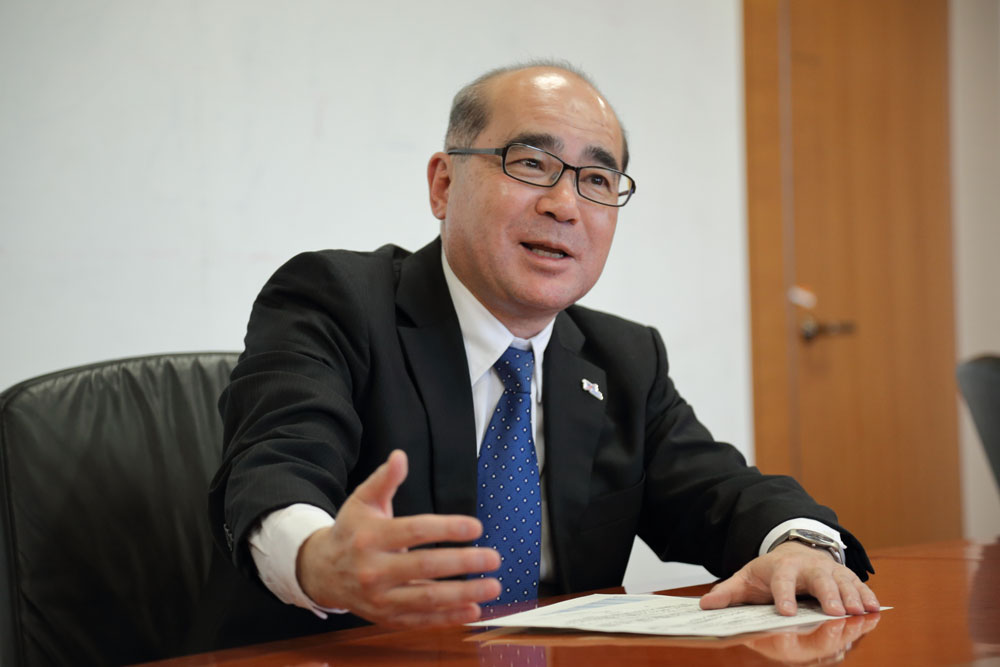
Ask the Experts: Tomoyuki Higuchi (The Institute of Statistical Mathematics)
Since 2011, Dr. Higuchi has served as the director of the Institute of Statistical Mathematics, the Research Organization of Information and Systems. An expert of Bayesian modeling, he is known for statistical modeling derived from real-world problems as well as research on data assimilation that combines mass data and theories behind simulative calculations. He leads various integrated research and works to foster the next generation of researchers. He serves on the ROIS’s board of directors and as an advisor to the Japan DataScientist Society. He received his bachelor’s degree in 1984 and his Ph.D. in 1989, both from the University of Tokyo.
Creating Big Data from Small Data
Data analysis techniques and AI technologies are constantly evolving and advancing. Where are we at in this journey, and what type of research projects are commanding the science community’s attention?
Kitsuregawa: The most interesting challenge we are tasked to tackle is the question of how to make small data big. For example, typhoons hit Japan typically 10 times a year, and this means we only get 100 incident data to learn from over 10 years. The question is, how we can draw the information that we need from the data on these 100 incidents? In other words, how do we data scientists do what we do when data is intrinsically lacking in certain fields? That’s the biggest challenge facing us right now.
Higuchi: Those are so-called rare events. They spur innovations and help make risk analysis more accurate. In mathematical statistics, researchers have traditionally used the “experimental design” method to prove a hypothesis through a limited number of experiments. But today’s science calls for an even more intentional system – a system that merges experiments, measurements and observations into a single process.
Kitsuregawa: Prof. Takaaki Kajita of the University of Tokyo, for instance, had very little experimental data, but his interpretation of the data has led to his discovery that neutrinos have mass, for which he received a Nobel Prize in 2015. This “interpretation” was a very sophisticated one and on a completely different level than AI’s pattern recognition and learning. But because Prof. Kajita was studying a phenomenon that occurs in accordance with the rules of physics, it was possible for him to trace it, step by step, to its causation. In most cases, you can’t do the same with what we see happening in this world. Take rare diseases as an example. It’s an area that we’ve been interested in, because unlike diabetes and hypertension from which millions of people suffer, we may only find one patient in Japan and another in England who have a certain rare disease. So, how do we go about finding a cure for a rare disease like that? Tackling such a problem requires us to bring together all talents and expertise and try different ideas.
Higuchi: In material data science, we may be able to determine the molecular bond and structure that gives substance a certain feature, but it is difficult to actually create that substance. This is the bottleneck in the practical application of advanced material data science. Researchers are experimenting with different approaches, such as simulating an experiment and searching for a pattern among its results. But when it comes to figuring out the process of creating a new material, experience still remains a more reliable tool than data science.
Kitsuregawa: In other words, just because a certain calculation method worked in identifying a material’s structure, that doesn’t tell you how to extend the method for finding a way to create the material. Also in natural language processing, the machine's ability to use big data for deep-learning is still limited. We need to figure out our next “card” that gets us through this bottleneck. In that sense, we are in a transitional phase.
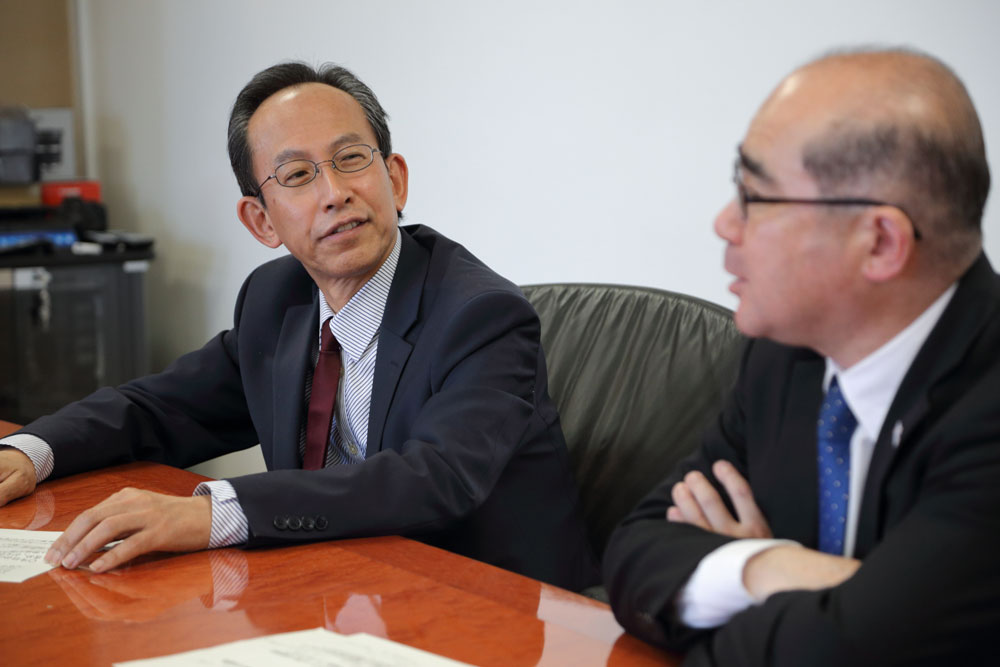
Getting Through Data Science’s ‘Bottleneck’
Data science is becoming an integral part of research in a wide range of fields from earth science to nanotechnology to health care to material development. While industry puts high hopes on data science as the key to innovations, directors Kitsuregawa and Higuchi say data science still has a long way to go before it can solve more complex problems.
Kitsuregawa: Science can effectively solve various problems, but there are certain areas where science can’t be of help, as I mentioned earlier. I believe we, the scientists, ought to academically identify those limitations, as well.
Higuchi: That’s right. I often explain this limitation issue through the concepts of interpolation and extrapolation. In data science, we basically make predictions within the bounds of existing data. That’s interpolation. Extrapolation is to go beyond the bounds and make predictions. Interpolation techniques have grown tremendously sophisticated over the years, contributing to the advancement of AI. The question now is how we are going to develop extrapolation techniques to provide solutions to problems. This is where we hit the brick wall. Another limitation of data science, currently, is that while it can show how things are correlated, it’s nearly impossible to demonstrate if there’s any causal relationship among them.
Kitsuregawa: Yeah, it’s impossible.
Higuchi: Right. I mean, you extrapolate data to predict the unpredictable, and it’s not possible yet to pinpoint exactly when and where an earthquake is going to happen, for example. Not, at least, until data science advances a little more and we have far better simulation models. The good news is that we are becoming better at quantitative risk analysis by making good use of high-speed computation and abundant data collected through observation networks.
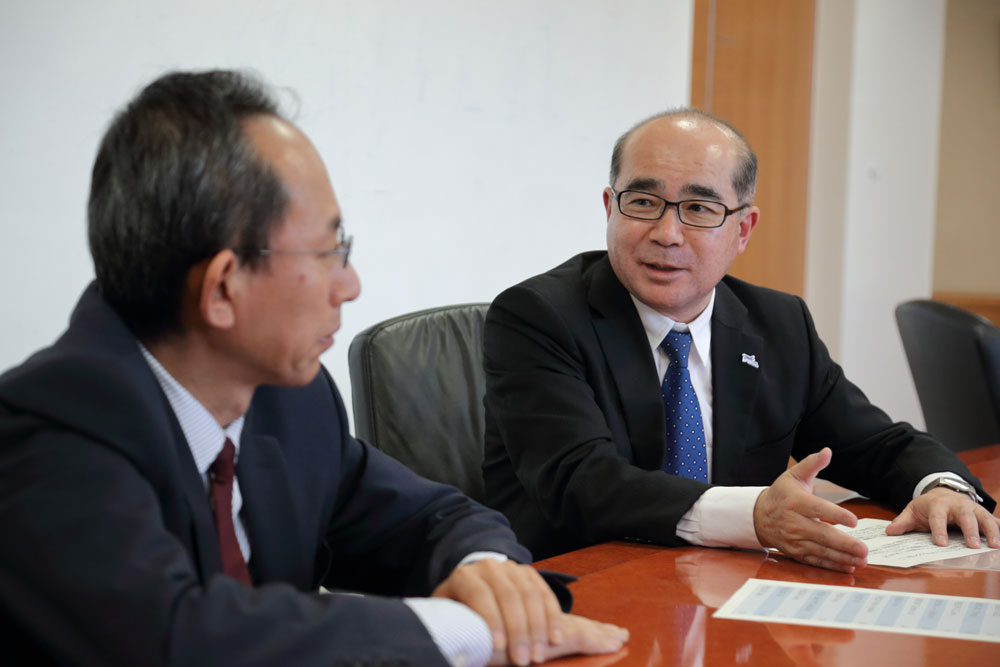
The Language of Data as Part of Basic Education
The big data era is here to stay, and data technology is expected to grow more sophisticated. So, how should we equip our children with the knowledge necessary to live in data society?
Kitsuregawa: Science and technology is advancing so much faster nowadays than in the past, following the “law of accelerating returns.” Eventually, it will reach the “singularity.” The biggest conundrum will be people’s slowness in digesting the fact and adapting to the new reality in everyday life. Just think about how long it may take to reform the Copyright Act to make it suitable for the internet era. You may adopt new technology to make society a less confusing place, but if people don’t understand its benefits, society would only grow more chaotic. I just wonder if there is a way to expedite the human recognition process.
Looking back on human history, a drastic transformation or the collapse of a culture has always occurred in its very final moment – equivalent of one last second versus an entire year. In hindsight, you can see there is a sequence leading up to such big changes, which is usually triggered by an unforeseen, peculiar human act, which then develops into a war, causing fear among the population. If this is any indication, if only technologies continue to advance without adequate time for people to appreciate what’s happening, it will sooner or later trigger major changes to our society. That’s my concern.
Higuchi: I feel the same way. This leads to the question of how we should educate the new generation. By the way, what are your thoughts on programming education in secondary schools?
Kitsuregawa: In Japanese schools, students learn Japanese first, English some years later and another language in college. I think the education of programming language should be given as much weight as any non-Japanese language, if not more. By having good English skills, you will be able to communicate with people from around the world and understand them, and that enriches your life. Programming language allows you to turn your idea into a tangible “thing.” In every IT course I teach, I always tell my students to bring something manmade to the first class. You will realize pretty much every product has a computer in it. This goes to show you that, without some knowledge of programming language, you won’t be able to make anything, and you may even have difficulty striking up a casual conversation with someone. In that regard, programming language should be part of the basic education for everybody. It is crucial that people begin learning how to make things at young ages.
Higuchi: We are also surrounded by data in today’s society. I hope future generations will grow up analyzing and interpreting data themselves to improve their daily lives. At the ISM, we have a program for secondary school students called ISM Data Science High School. It may sound like a place that teaches complex machine learning and analytical methods, but that’s not our focus. We want to provide the students with learning opportunities to figure out on their own that how they select a problem to tackle, and what angle they take to solve it, matter the most in data science.
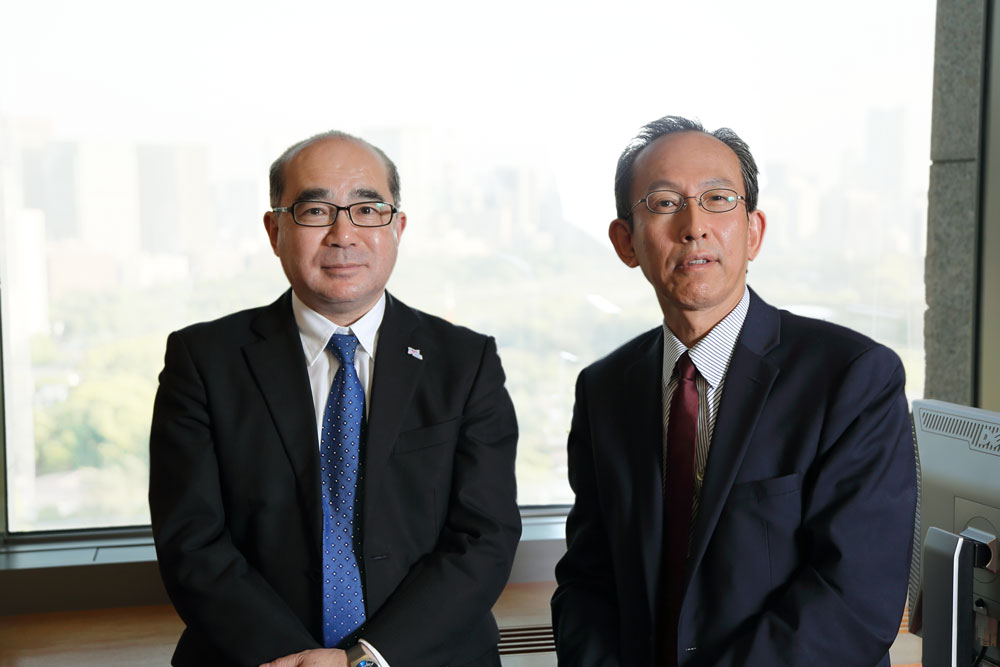
Interviewer: Rue Ikeya
Photographs: Yuji Iijima unless noted otherwise
Released on: January 21, 2019 (The Japanese version released on June 11, 2018)