Changing Patients' Lives with Data Science
Saving patients’ lives isn’t just the responsibility of medical and healthcare professionals any longer. It takes a village to provide the best care possible, and playing increasingly important and larger roles in it are data scientists and people in the associated fields. This trend precipitated the recent openings of the two national data research centers – one at the National Institute of Informatics (NII) and the other at the Institute of Statistical Mathematics (ISM). The Research Center for Medical Bigdata at the NII opened in November 2017, followed by the Research Center for Medical and Health Data Science at the ISM’s opening in April 2018. Both the NII and the ISM are part of the Research Organization of Information Systems (ROIS). The two new research centers work to muster all the information technology (IT) and statistical knowledge and resources available in Japan while promoting interdisciplinary collaborative research projects as members of the Inter-University Research Institute Corporation. Well-managed data infrastructures can propel scientific discoveries and innovations, and so does superior analytical capacity, such as that of artificial intelligence (AI). Now that the newly opened research centers aim to deliver both, how will that help drive forward Japan’s medical research and health care? The directors of the two centers will share their visions for future applications of data science in medicine.
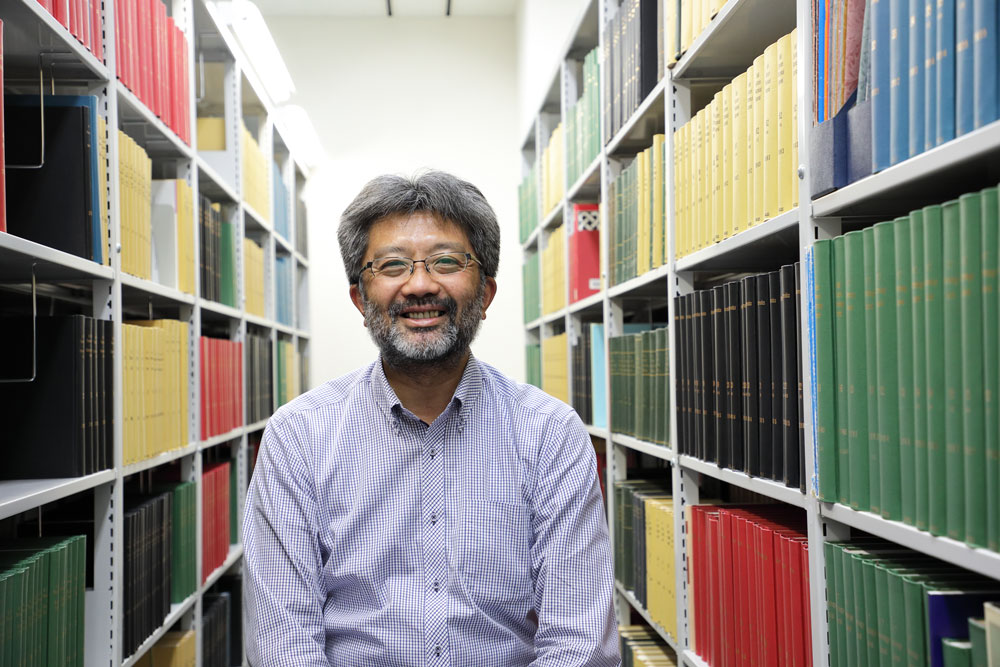
Ask the Experts: Shin'ichi Satoh (National Institute of Informatics)
Professor at the National Institute of Informatics since 2004 after serving as associate professor at the institute since 2000. Expert in image and video analysis-based information search and knowledge discovery. Previously worked at Carnegie Mellon University as visiting research associate from 1995 to 1997. Holds a Ph.D and undergraduate degrees in engineering from the University of Tokyo.
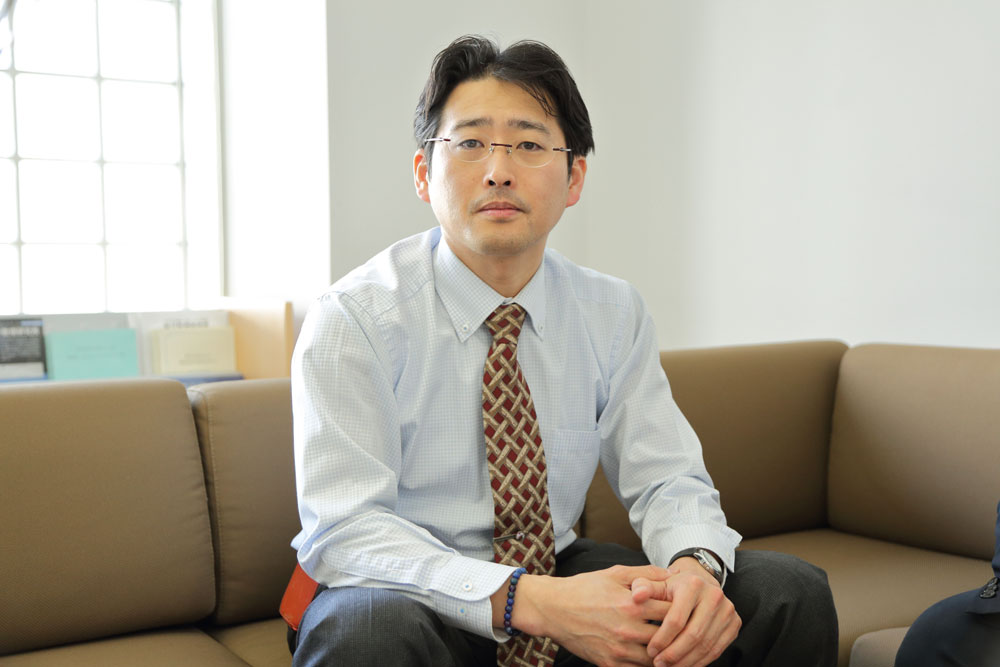
Ask the Experts: Yoichi M. Ito (The Institute of Statistical Mathematics)
Professor at the Institute of Statistical Mathematics. Specializes in biostatistics with a focus in genomic and statistical analysis and provides consultation on the topics. Has served as an expert member on the PMDA, a reviewing board for new drugs, since 2009. More recently, working to analyze clinical trial data through the lens of efficiency to develop ways to improve data management. Holds a Ph.D. in health sciences from the University of Tokyo.
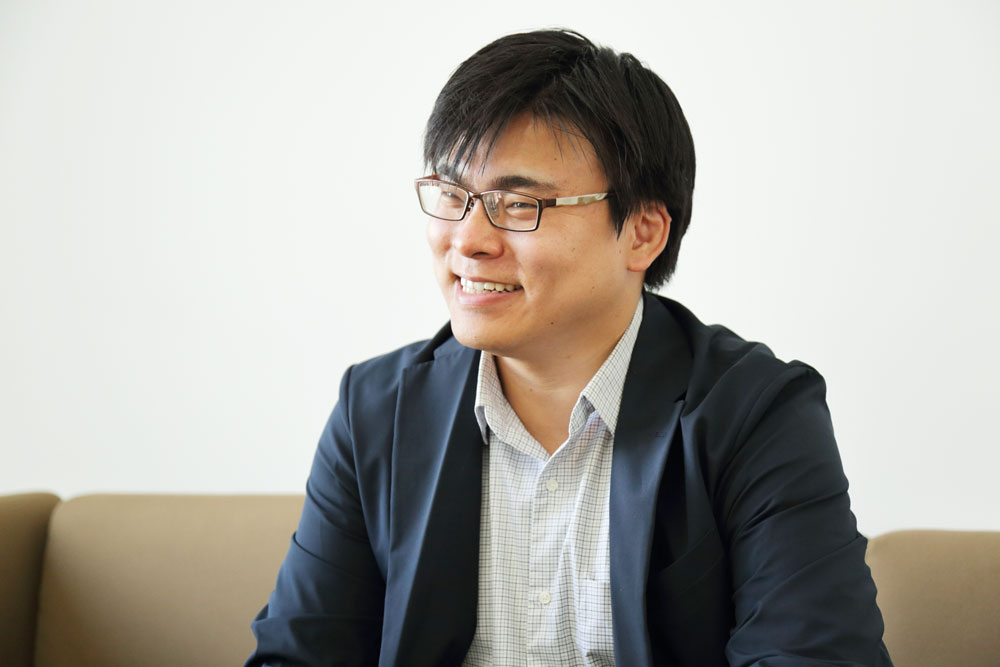
Ask the Experts: Hisashi Noma (The Institute of Statistical Mathematics)
Associate professor at the Institute of Statistical Mathematics. Appointed to the current position after studying biostatistics at the Graduate School of Medicine, Kyoto University and serving as the ISM’s assistant professor. An expert in the fields of medical statistics and public health, he hopes to “contribute to the advancement of medicine and health care through research and education.”
Bringing the Best of the Best in the IT World to Help Physicians
The National Institute of Informatics (NII), which is home to the newly opened The Research Center for Medical Bigdata, is Japan’s only general research organization solely focused on information technology (IT). The NII became the Japan Agency for Medical Research and Development (AMED)’s partner organization in 2017 and has since worked with four academic societies – the Japan Gastroenterological Endoscopy Society, the Japanese Society of Pathology, the Japan Radiological Society, and the Japanese Ophthalmological Society – to develop the IT infrastructure for the medical and health care communities. The collaborative efforts include image analysis, deep learning and work toward the development of AI.
The Research Center for Medical Bigdata now leads these efforts. The center has about 10 research team members, some of whom are enlisted from such outside organizations as the University of Tokyo, Nagoya University and Kyushu University. The members also include some young post-doctoral researchers. They are among the brightest in their own fields, but most of them don’t have any experience in analyzing medical images.
“I, for one, am a researcher specializing in image analysis and deep learning and had never dealt with medical images before,” said Prof. Shin'ichi Satoh of the NII, who serves as the director of the Research Center for Medical Bigdata. “The thing is, we can analyze medical images. Though we don’t know what we are looking at, the artificial neural network that we trained, which is a type of AI, does know what to do,” he said.
Until recently, scientists in the field of medical image analysis were trying to create computer programs for data processing that reflect their medical knowledge, according to Prof. Sato.
“There has been a ‘shift in the tide,’ if you will, as we became capable of feeding an astronomical amount of data to a machine to make some sense of it,” he said. “It’s been only a year and several months since our collaborative research began, but we are already seeing a lot of successes with the use of machines. For example, very basic artificial neural networks can quite accurately diagnose diabetes and glaucoma, particularly when using fundus images as a diagnostic tool. These diagnoses are actually challenging ones for physicians to make,” he said.
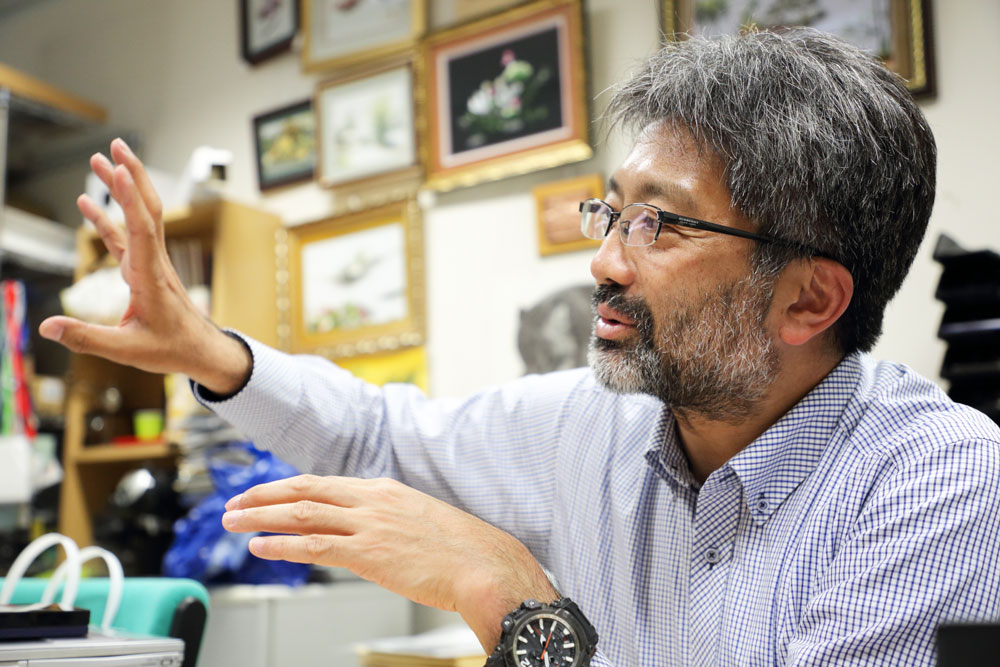
A Turning Point in the ‘Game of Computer Image Recognition’
Prof. Sato pointed out there have been three major “waves” that pushed forward the research on artificial neural networks over the years.
“The first wave was the emergence of a neural network that mimicked that of the human brain. This was technically a single-layer network capable of learning how to use randomized linear functions. It proved that machines can learn from inputs and became the framework for machine-learning. The second wave was the arrival of so-called neuro-fuzzy. This was a little more complex network with two to three deep-learning layers,” Prof. Sato said.
“Then, in 2012, a revolutionary algorithm mimicking the human brain’s neuron network became available. Its ability to learn and think far exceeded that of any other algorithm that existed at the time. This spurred interest in leveraging the technological advancement and availability of big data to make discoveries. This is the third and latest wave in the history of neural network inventions,” he said.
The revolutionary computer vision algorithm was built by University of Toronto professor Geoffrey Hinton – who is known in the research community as the “Godfather of AI” – and his students for a competition in which they participated. The team gave the large, deep convolutional neural network 1.2 million images to train it to recognize 1,000 different types of objects.
“The team’s research paper gave researchers an understanding of how much data a machine needs to do its job and at what level of accuracy. It became a turning point in the game of computer image recognition,” Prof. Sato said.
“Before this, what objects a machine should recognize and how it should be done were all determined by humans. What sets Prof. Hinton’s neural network apart from the rest was that it was designed to teach itself from scratch what to do and how,” Prof. Sato said. “When you train this type of network by giving a huge number of images containing 1,000 different objects, it does a superb job recognizing objects in newly introduced images. Unfortunately, we don’t know what the machine learned, but it clearly can carry out very efficiently what we humans used to rack our brains to do.”
Prof. Hinton’s algorithm had eight learning layers. Nowadays, deep-learning machines with 100 to 200 layers are commonplace, according to Prof. Sato.
The theoretical analyses that provide the foundation of deep learning have remained unchanged for the past three decades, Prof. Sato said. But, what you can do with artificial neural networks, especially with computer vision recognition, has drastically improved over the years, and researchers have now had experiences to better understand the possibilities as well as the limits that artificial neural networks present, he said.
At the Research Center for Medical Bigdata at the NII, researchers are working toward finding efficient ways to use computer image recognition technologies for analysis of medical big data.
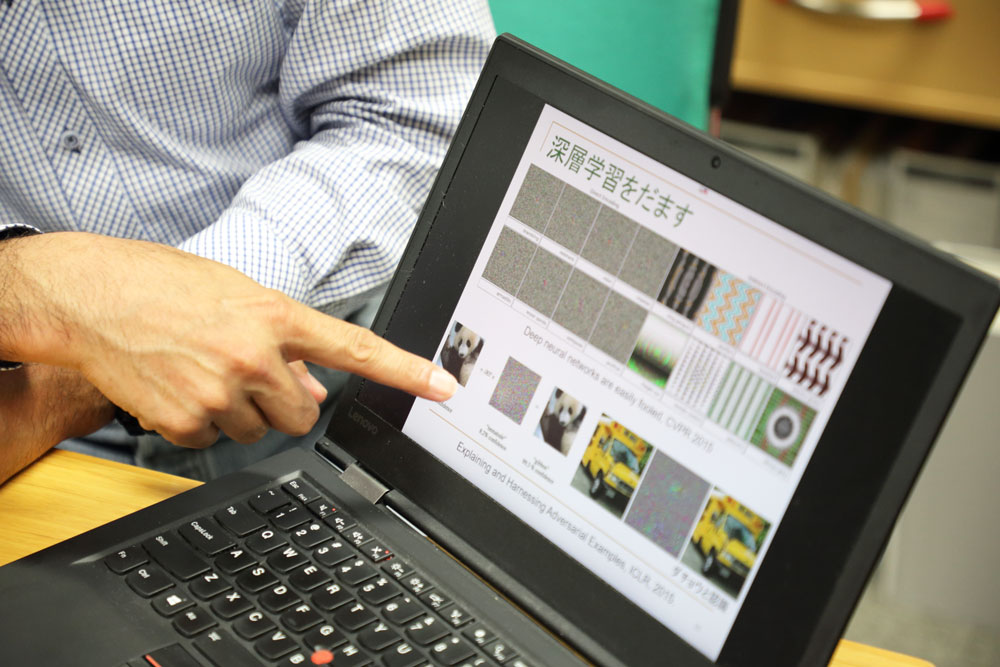
Making Doctors’ Lives Easier
Prof. Sato believes image recognition algorithms can help reduce physicians’ workload. To achieve this goal, his team is compiling “correct diagnostic interpretations” of image data that can be used to train the machines.
“If you were analyzing image data to correctly recognize cats and dogs, anyone could do it. But medical analysis requires physicians’ expert knowledge. Data has to be looked at by physicians, and they need to tell you what the right interpretation is. This defeats the purpose of making physicians’ lives easier,” Prof. Sato said. “So, we use self-learning algorithms to narrow down the scope of data that requires physicians’ judgement and request doctors to attach correct interpretations to it. This considerably reduces physicians’ workload. But, I think we can and should make the algorithm even more physician-friendly,” he said.
Algorithms could also assist physicians in other ways to improve the quality of medical care.
“In the near future, we may have an image analysis system designed to provide support to physicians. The system could prevent physicians from overlooking important information or offer an independent second opinion. It could also diagnose minor illnesses, so the physicians can use their time to focus on other illnesses that are more difficult to diagnose,” Prof. Sato said. “It’s our dream to develop an advanced AI algorithm that has the judgement of experienced physicians. If we create that, we can then make it available in remote places like islands. It’s a program, and so you could easily replicate it, as well,” he said.
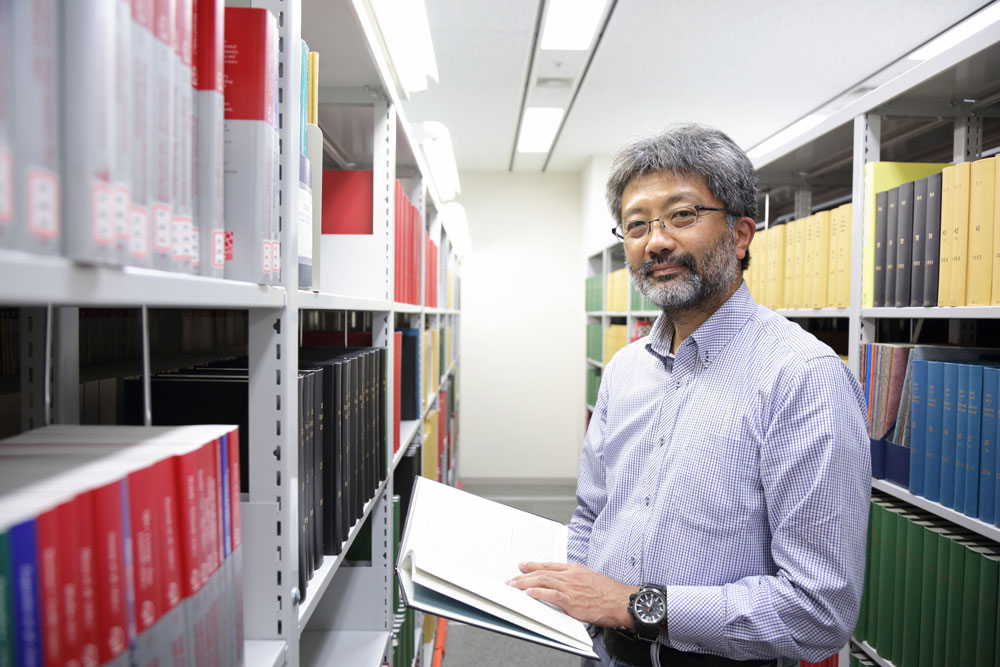
Fostering Data Scientists in University Medical Departments
In the meantime, the Research Center for Medical and Health Data Science, which recently opened at the Institute of Statistical Mathematics (ISM), aims to bring together medical statistics experts and build different types of intelligence infrastructures to prepare the country for medical and health care in the coming data age.
“More than 60 universities across Japan have medical departments, and they all critically lack biostatisticians. The center’s missions include fostering the next generation of data scientists with the knowledge and skills required to assist the medical community, as well as providing training for literacy in medical statistics,” said Prof. Yoichi M. Ito of the ISM, who serves as the director of the center.
The center offers four courses a year on education of medical statistics. Five publicly accessible seminars have already begun as part of these courses, and a symposium organized to celebrate the center’s inception was also held on May 28, 2018.
“The foundation of this center would help raise the level of enthusiasm for data science in the medical and healthcare community, and the change was palpable,” said Dr. Hisashi Noma of the ISM, who serves as vice director of the center and directed the symposium’s operation.
Prior to the center’s opening, the ISM called for the formation of the Medical and Health Data Science Research Network to promote statistical education. More than 70 institutions, including universities and hospitals, quickly answered the call, Dr. Noma said.
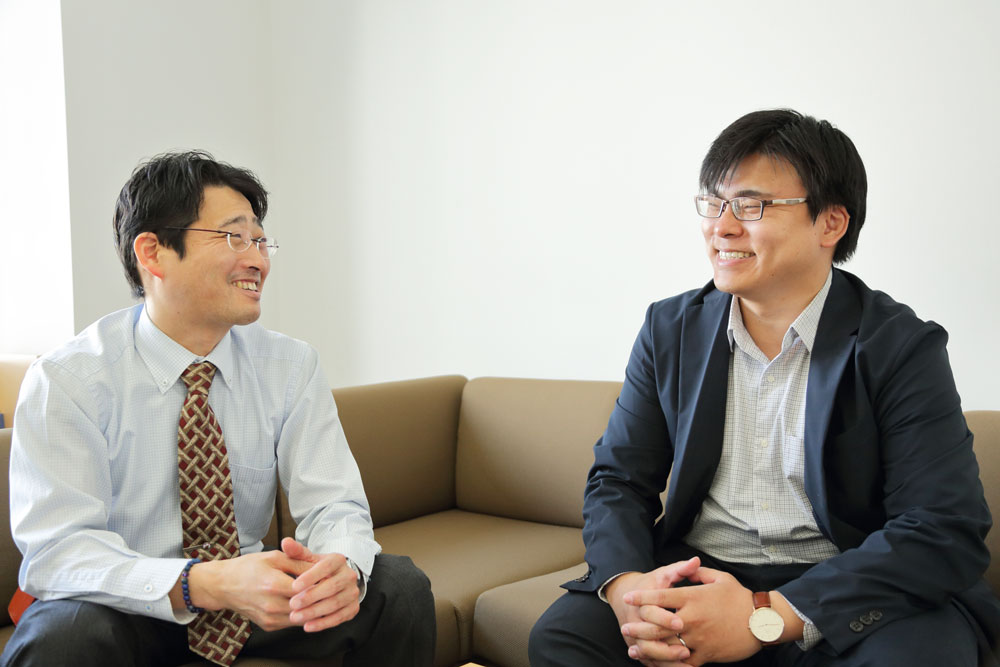
Rigorous Evaluation of Clinical Trials from Statistical Science’s Standpoint
Evaluation of clinical trials for new drugs is another area where Prof. Ito believes the Research Center for Medical and Health Data Science can make contributions.
“As you know, statistics has always played a critical role in life science, particularly pharmaceuticals. I mean, statistics is an essential tool for understanding the effectiveness and safety of a new drug when evaluating the result of a clinical,” said Prof. Ito, an expert of clinical trial evaluation who has years of experience in helping design clinical researches as a statistical consultant.
“The human body has biologically complex systems and exhibits very different responses to drugs than other species do. Over the years, we have seen human subjects in some clinical trials experience totally unexpected side effects to drugs that had proved safe in mice, dogs, and even monkeys that are biologically more similar to humans. To understand whether a new drug has any therapeutic benefit or if it’s safe for humans from the result of clinical trials, we must apply statistical science to come up with an exact margin of error and scientifically evaluate the data. This is where statistical skills come in,” Prof. Ito said.
“Design methods for clinical trials are also improving every day, and so we need to keep pace with new methodologies for statistical analysis. This is a highly specialized field in which every step is intentional and calculated – which makes a sharp contrast with analysis of big data,” Prof. Ito said.
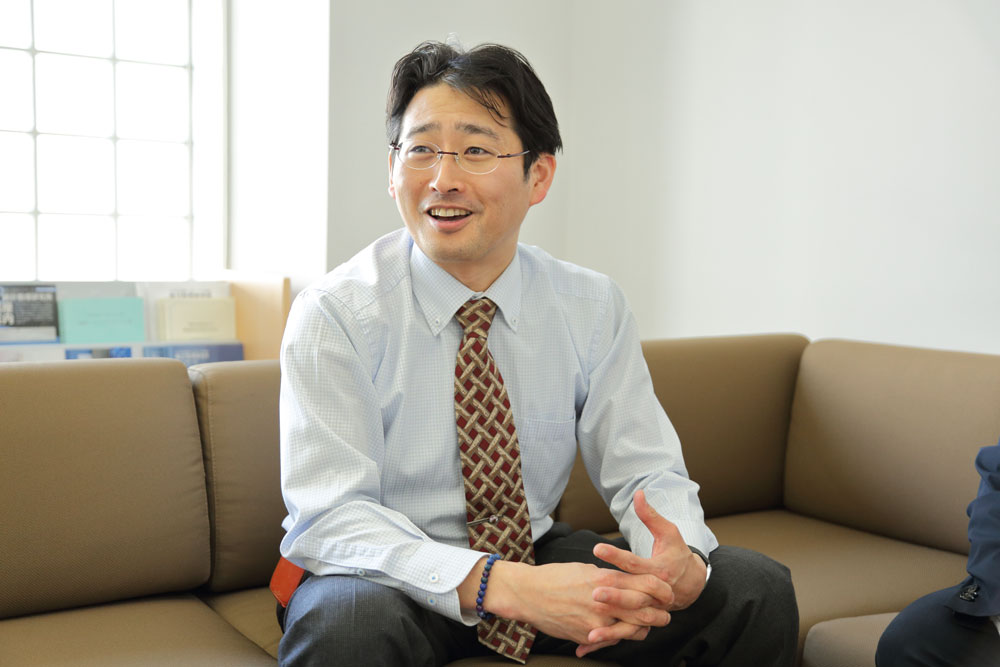
Accurately Estimating Harmful Environmental Factors
Epidemiology, the study of distributions and determinants of human health and diseases, is another area in which statistical science is making a big difference. The history of epidemiology goes back to the 19th Century England when scientists began researching what caused the spread of cholera and other infectious diseases and how to prevent them. Since then, it has evolved into the study of medical care and health in general as disease patterns have diversified in modern society. More specifically, epidemiologists refer to those who make scientific assessments of the health impact of such environmental factors as cigarette smoke, hormone-disrupting chemicals and air pollutants, using observational studies of humans as their basis, according to Dr. Noma, who has worked on epidemiological research projects since college. “The problem is that we have no way of controlling those harmful environmental factors like you would in normal scientific experiments. We rely on statistical methodology to precisely determine how and from which subjects we should collect data and whether other factors are involved,” he said.
Epidemiological experts particularly try to look at data through the lens of statistical science to minimize the effect of biases.
“Measuring biases is very difficult. It’s just not possible to set ‘perfect’ questions that produce no biases in evaluation,” Dr. Noma said. “But, because you are evaluating public health risks, even though you know what you have includes a bias, you have to take action in response to it to protect the public. Then you again need the help of statistical science to figure out what an effective action would be.”
Epidemiology began a long time ago, way before statistical science did. In recent years, the methodologies used in epidemiology started becoming more refined. Methodologies didn’t originate in clinical trials, which is my specialty, but the fields of epidemiological statistics and life science statistics, such as what you obtain in clinical trials, are crossing over, spurring advancement of methodologies,” Prof. Ito said.
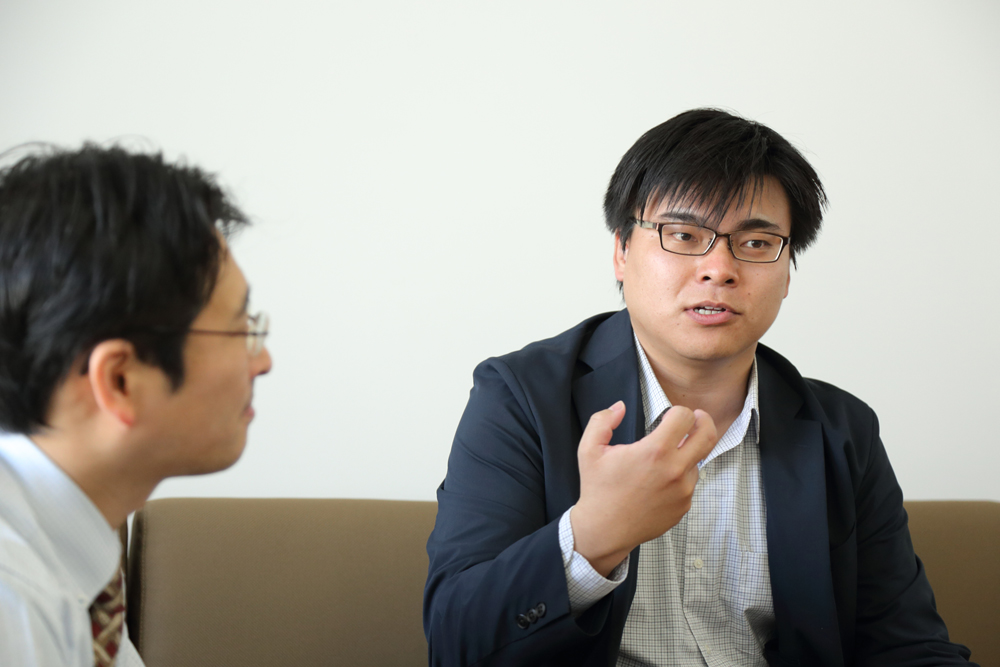
Building New Methodologies from the Base
The Research Center for Medical and Health Data Science has six ongoing research projects, and one of them is called, the “Project to develop methodologies for clinical researches and evidence synthesis.” The project brings together the center’s dual interest in developing novel methodologies and promoting big data analysis to make “precision medicine” available in daily medical practices. Efforts to advance research on precision medicine are taking place around the world, including in the United States where former President Barack Obama announced the Precision Medicine Initiative during his 2015 State of the Union address.
“For example, thalidomide is infamous for bringing on horrible side effects in patients worldwide during the 1950s and 1960s. But, in 1999, a group of scientists in the U.S. proved that the drug that prevents angiogenesis, can effectively be used to treat myeloma, resulting in the approval as an anti-cancer agent in Japan in 2008. Through data analyses that use data science methodology, researchers are now finding out that the nature and degrees of a drug’s effects vary from patient to patient,” Prof. Noma said.
“Let’s say you have cancerous cells removed from a patient. You measure all sorts of things for comprehensive analysis of genetic and molecular-level information, and you will end up with more than several million dimensions of data. This is what you call omics data. Omics data has become available, partly thanks to the considerable advancement of measurement techniques in the past two decades,” Prof. Noma said. “Using our new statistical methodologies to analyze patients’ omics data in connection with thalidomide’s effects, for example, it becomes clear that those who were treated with the drug have better prognoses than patients who didn’t take the drug. In addition, thalidomide affects individual patients differently, depending on whether their molecular cells show certain gene expression patterns. By applying newer methodologies to the analysis of omics data, you can gain such detailed insights,” he said.
"With research projects beginning to leverage big data in more diverse ways, methodology for data analysis is needing to change and diversify, as well. The Research Center for Medical and Health Data Science strives for fundamental research to help fulfill this demand, conducting research on such topics as the use of machine learning technology for exploratory analysis of genetic data, as well as the theoretical development of classical statistics," Prof. Ito said. “It is our highest priority to establish an analysis model to offer patients accurate prognoses, rather than to get a complete picture of a group of patients. This is the most urgent task for us. It’s also an intriguing challenge for us to tackle.”
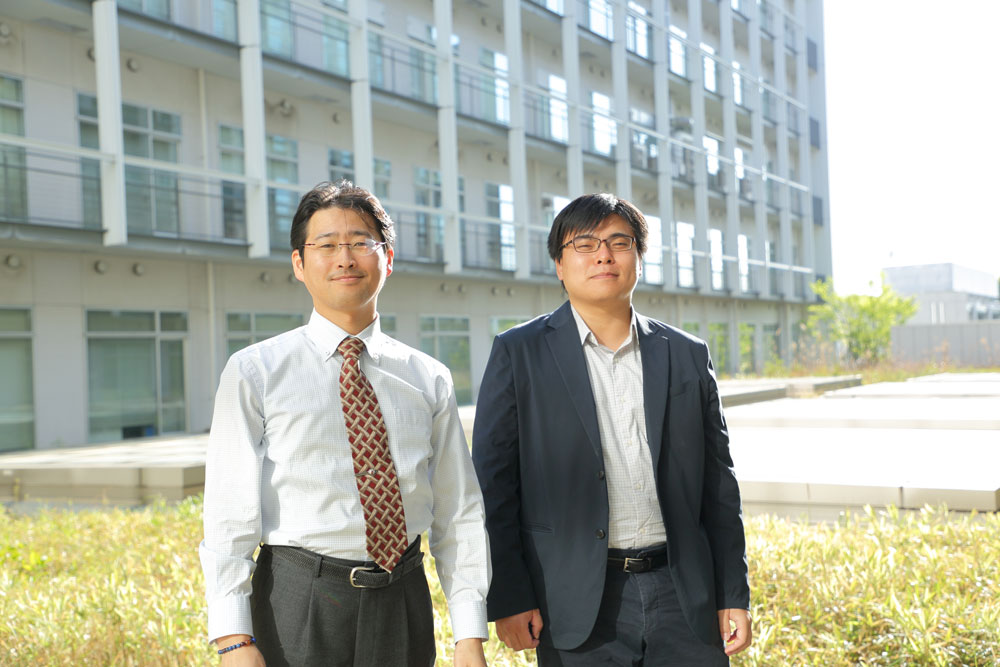
Interviewer: Rue Ikeya
Photographs: Yuji Iijima unless noted otherwise
Released on: December 18, 2018 (The Japanese version released on May 10, 2018)