Reinventing the Process of Making Things
Decades after “Made in Japan” became synonymous with high quality, the country is pushing for scientific breakthroughs that would help industries redefine excellence in manufacturing. The initiative focuses on leveraging data science and artificial intelligence (AI) for manufacturing, following in the footsteps of the U.S., Germany and many other nations in Europe and Asia, where similar movements have already been underway. In hopes of galvanizing ongoing efforts in Japan, the Research Organization of Information and Systems (ROIS) established two centers last year within the existing institutes under the ROIS’s umbrella – the Data Science Center for Creative Design and Manufacturing, launched at the Institute of Statistical Mathematics, and the Global Research Center for System Design and Mathematics, launched at the National Institute of Informatics. So, what’s the vision of Japanese leaders in this scientific frontier? And how could they take manufacturing to a whole new height? The scholars who lead the two new research centers will spell it out for us as they explain about the projects that they lead.
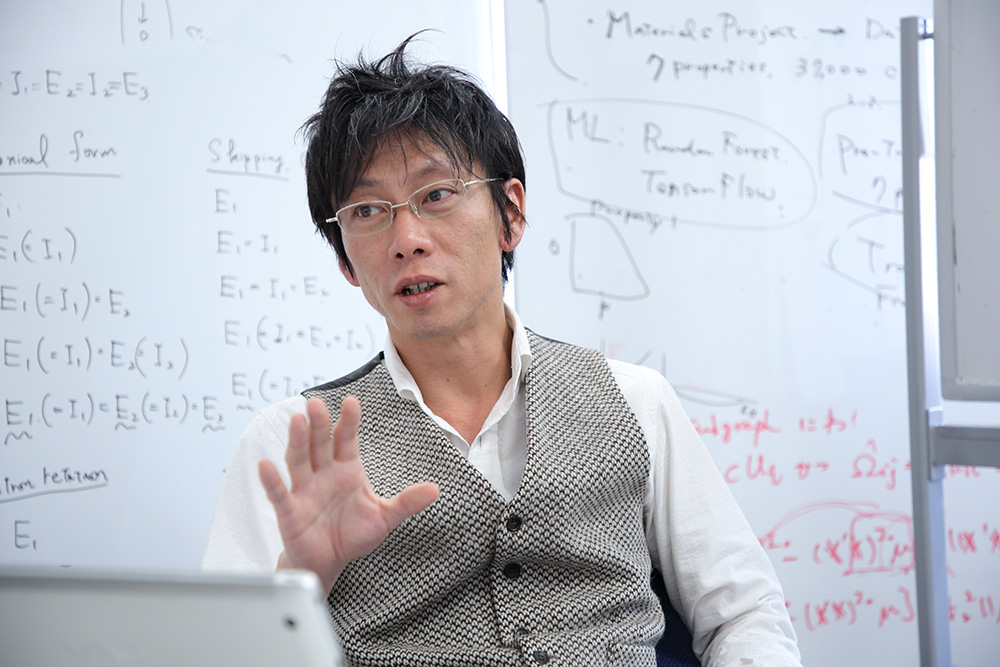
Ask the Experts:Ryo Yoshida (The Institute of Statistical Mathematics, ISM)
Dr. Ryo Yoshida, professor for Department of Data Science at the Institute of Statistical Mathematics (ISM), has served as the director of the Institute’s Data Science Center for Creative Design and Manufacturing since the center’s opening in July 2017. After receiving his doctorate degree in Statistical Mathematics from the Graduate University for Advanced Studies in 2004, he worked as a Project Assistant Professor for the Human Genome Center at Institute of Medical Science, the University of Tokyo – a position he has maintained after joining the ISM in 2007. In addition, he serves as a specially appointed researcher for National Institute for Materials Science. Dr. Yoshida has the experience of using his expertise in data science for research work in both biology and materials science. He plays leadership roles in several cutting-edge research projects, including the Japan Science and Technology Agency (JST)’s the “Material Research by Information Integration” initiative.
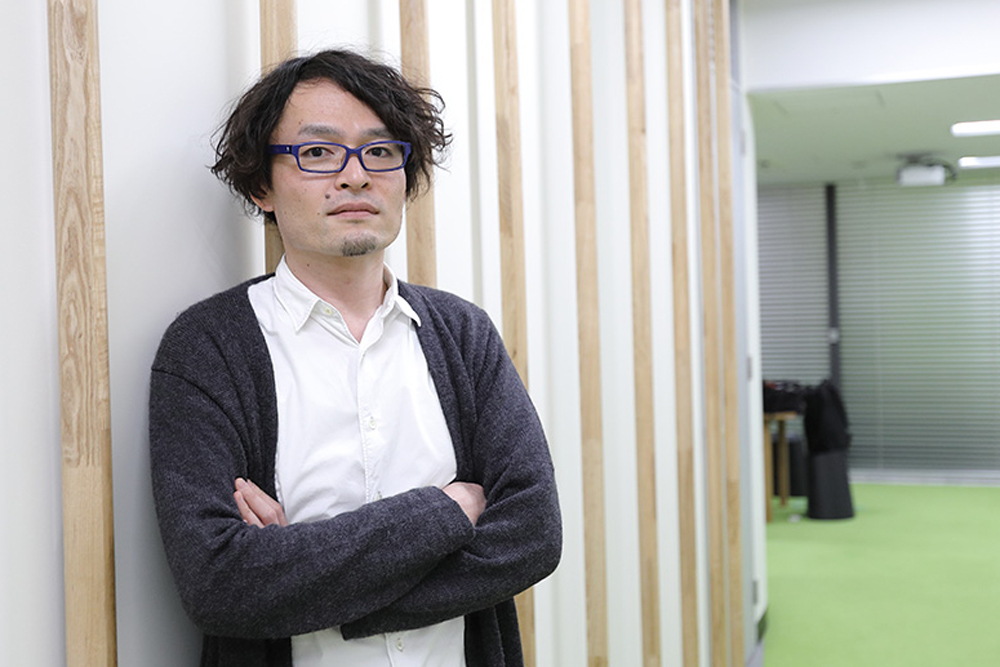
Ask the Experts: Ichiro Hasuo (National Institute of Information, NII)
Dr. Ichiro Hasuo, associate professor for the National Institute of Informatics (NII)’s Information Systems Architecture Science Research Division, has served as the director of the Institute’s new Global Research Center for System Design and Mathematics since July 2017. With his expertise in theoretical computer science, he is particularly interested in such research topics as system verification, programming language theory, information processing, and mathematical structures in information science. He is a 2002 graduate of the University of Tokyo and received his doctorate in computer science from Radboud University Nijmegen in the Netherlands. Before joining the NII in April 2017, he served as associate professor for the Graduate School of Information Science and Technology at the University of Tokyo as well as visiting associate professor for the Research Institute for Mathematical Science at Kyoto University. Since 2016, he has led the Hasuo Metamathematics for Systems Design project as part of the Japan Science and Technology Agency’s Exploratory Research for Advanced Technology (ERATO) program.
Materials Informatics: Trading Test Tubes for AI
One of the study fields that are increasingly attracting the attention of both the academic and manufacturing communities is materials informatics. Public interest in the field grew following the 2011 launching of the Materials Genome Informatics Initiative by the U.S. government under the leadership of the former president Obama.
Researchers used to go through countless trials and errors in wet labs to develop new materials for products. When things do or don’t work out in the ways hypothesized, that would shed that much more lights on the complex relationships between material chemistry and physics. Researchers would use the knowledge to conduct additional experiments and inch toward discovering ground-breaking materials. This long-standing empirical approach to inventing materials is becoming a thing of the past, now that materials informatics allows researchers to make discoveries without compiling direct evidence to get there, according to Prof. Ryo Yoshida of the Institute of Statistical Mathematics (ISM).
Materials informatics refers to a field of study that harnesses the power of big data and AI to rapidly discover novel materials that could be created. Before conducting direct experiments and physically measuring values, researchers would put existing big data as well as interpolative data derived from it through algorithms and let AI identify patterns in the data. AI uses the patterns to predict how proposed materials would behave in the real world and/or what properties a material need to be made of to offer desired characteristics and features.
This drastically accelerates the speed of material innovations. It also allows researchers to create something that they never imagined or have considered as far-fetched, said Prof. Yoshida, who serves as the director of the ISM’s Data Science Center for Creative Design and Manufacturing.
For example, the Power conversion efficiency of photovoltaic cells is currently limited to 11 percent maximum, Prof. Yoshida said, as he showed a graph with dots representing the efficiency of cells made of different materials.
“The vertical line in the middle is the maximum efficiency we can attain with the existing scientific knowledge and materials. The area to the right is the ‘uncharted territory.’ Our job is to find materials that fall in this territory,” Prof. Yoshida said. “We want materials with features and characteristics that enable cells to achieve far more than 11 percent of efficiency,” he said.
“Over many decades, material science gradually advanced, pushing the middle line in the graph to the right. Now, we have the opportunity to expand this dotted region at exponential speeds by taking advantage of the cutting-edge data science technology. This will definitely be a boon to scientific and industrial advancement.”
Prof. Yoshida pointed out that data-gathering and identification of patterns with the use of machines is the essence of data science.
“In data science, we, the researchers don’t have to come up with any theories. We just need to have the machines analyze data to recognize hidden patterns and identify the theory behind them with inverse problem methods. The computer uses the theory to build a virtual material with the structure and properties that are necessary to provide desired features,” Prof. Yoshida said.
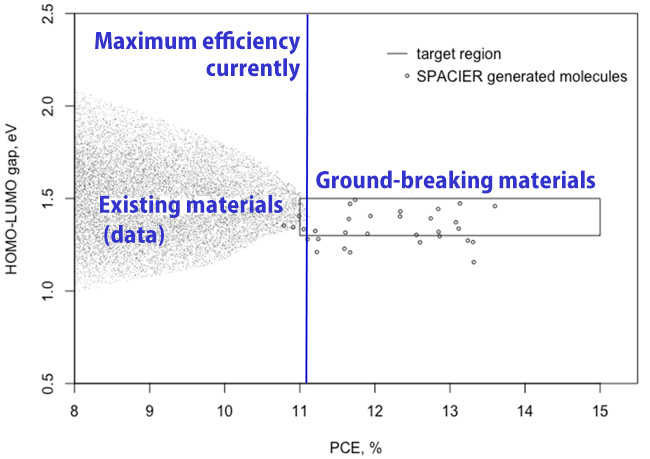
Getting Mileage out of Data Science with Extrapolative Data
Prof. Yoshida has particularly been interested in the use of extrapolated data to enhance the machine’s ability to create a virtual material far, far outside of the current realm of possibility. That’s because the computer needs more data than currently available to theorize advanced materials on it.
“You can make only so much prediction from data. To explore outside the ‘predictable zone,’ you would need additional data. That’s the limitation of data science,” Prof. Yoshida said.
“Data science has never played crucial roles in any Novel Prize-level, ground-breaking scientific discoveries. Technological advancements have always come from direct experimentations and theories. When I think about what contributed to my research successes thus far, data science accounts for 5 to 10 percent of it,” Prof. Yoshida said.
He noted that data science has increasingly had artistic applications in recent years, such as machine production of videography and music and designing of anime characters. However, whatever the field you are in, the availability of data or the lack thereof still dictates what you can do with data science,” Prof. Yoshida said. “Therefore, if we wanted a machine that can make advanced predictions – those that go far beyond the predictions based on the knowledge and experiences humans currently have, then the machine needs to be capable of extrapolating data to make up for what’s missing.”
This idea inspired Prof. Yoshida’s research team to develop an algorithm called SPACIER.
“SPACIER sets up a virtual materials research laboratory inside a computer. It designs experiments and conducts simulations in the virtual lab to extrapolate data,” Prof. Yoshida said. “The machine solves inverse problem to enhance its predictability, so it can find materials beyond the existing ‘predictable zone.’ You can repeat this extrapolation process through the algorithm to gradually push the limit.”
Theoretically, he said, it’s possible to keep using the algorithm to generate more and more data, further expanding data distributions. It’s also possible to modify SPACIER for general applications in other fields, including such a purpose as structural designing of industrial products, Prof. Yoshida said.
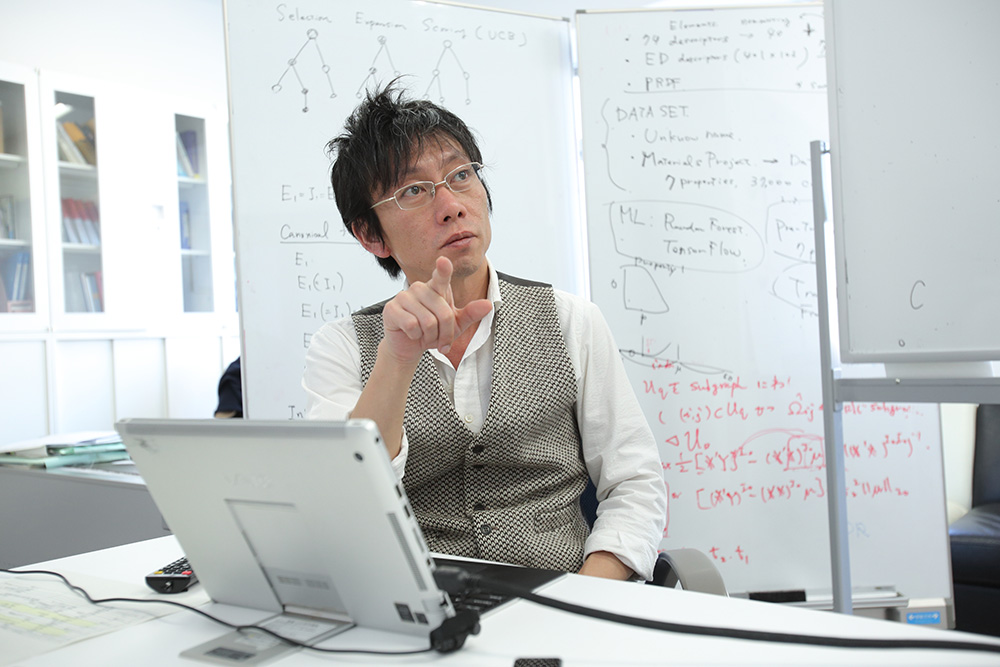
Collaborations and Blending Tools Key to Reaching the Goal
While combinations of algorithm, AI and extrapolative data may sound like a magic wand for inventing things, Prof. Yoshida cautions that there’s still a way to go before practical applications become routine. Don’t get too excited about machines like AlphaGo Zero, the robot that gets better at playing an Asian board game known as Go by learning from its own actions to outdo itself.
“We’ve only begun making ‘super creative’ machines that internally generate data to serve its purpose. There are many technological challenges to overcome to perfect the mechanisms,” he said. “At least for now, experiments in virtual labs alone cannot suffice to meet all industrial design and development needs. It’s important that we create formulae for turning ideas into products by blending physical experiments, theories, computational research and algorithms.”
Prof. Yoshida’s mid-range vision calls for robust machine learning with the use of data and theories from direct experiments. A machine would feed on existing data and take back what’s come out of the algorithm after theories are applied to it. But, how would he convince the manufacturing industry to adopt the new methodology?
“Show. Don’t tell,” Prof. Yoshida said. “We want to send the message out that the methodology does work and that data science can be the most powerful ally in innovating products under the right partnerships. I intend to bring this message to those on the front line of industrial development research over the next few years.”
As part of this initiative, the Data Science Center for Creative Design and Manufacturing plans to collaborate with six corporations this year for data scientists training. The center hopes to work with many more companies in the years to come, Prof. Yoshida said.
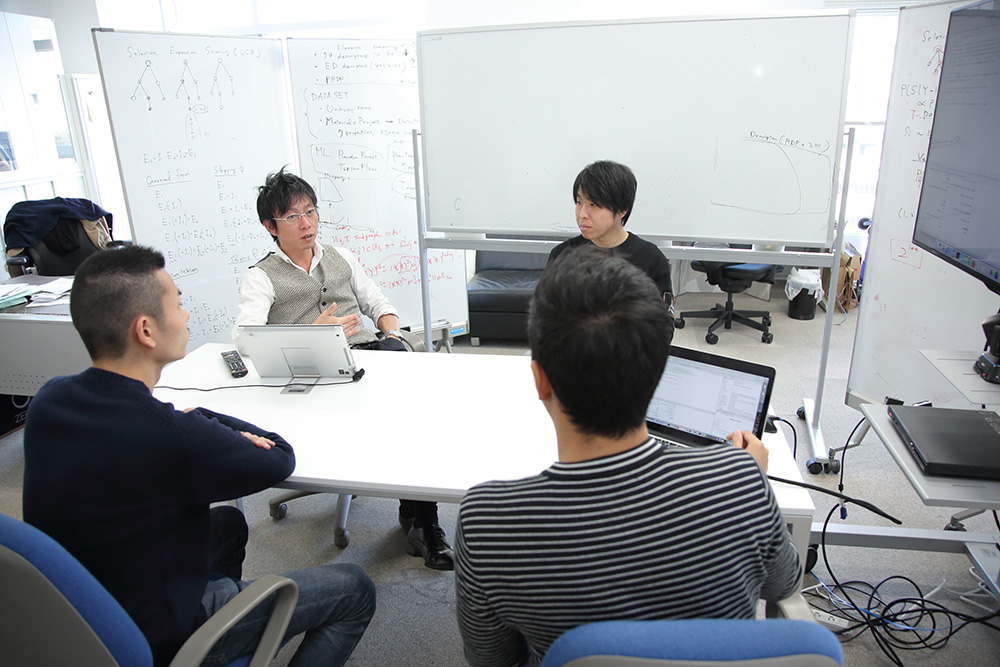
New Era of Quality Assurance Begins with Self-Driving Cars
Another area in information science that a group of ROIS researchers is trailblazing to advance manufacturing is called “category theory.” This effort stems from the fact that most machines today are computerized, necessitating manufacturers to reconsider how to carry out quality assurance, according to Prof. Ichiro Hasuo of the National Institute of Informatics (NII)’s Information Systems Architecture Science Research Division.
“Just think about self-driving automobiles,” said Prof. Hasuo, who also serves as the director of the NII’s Institute’s new Global Research Center for System Design and Mathematics. “Even the most experienced auto makers’ considerable know-how can’t be of enough help when it comes to performing quality control and assurance in this nascent field of self-driving automobile technology. Manufacturers are understandably unsure about how to navigate these uncharted waters. Academia are equally confused about which discipline’s responsibility it should be to address this issue.”
That’s where Japan Science and Technology Agency (JST)’s Exploratory Research for Advanced Technology Metamathematics for Systems Design Project (ERATO MMSD project) comes in. Directed by Prof. Hasuo, the ERATO MMSD project aims to expand the formal methods – mathematical methods to structure software systems – with the use of category theory, so formal methods can be applied to cyber-physical systems (CPS) as well as physical products. The project places a particular focus on automobiles.
“It began at the right timing,” Prof. Hasuo said of the ERATO MMSD project, which was launched in October 2016 and is now housed at the Institute’s new Global Research Center for System Design and Mathematics, for which he serves as the director.
“Industrial equipment used to be kinetic machines, and their stabilization systems were based on the control theory. But nowadays, nearly every machine has a computerized control system installed in it,” Prof. Hasuo said. “Generally, simpler machines are linear systems, meaning their response to outside forces is straightforward and predictable. If you give it twice as much force, the output would also be twice as much. Computerized control systems for automobiles and other large, complex systems don’t act that way.”
So, how do they act? The ERATO MMSD Project will answer the question, Prof. Hasuo said.
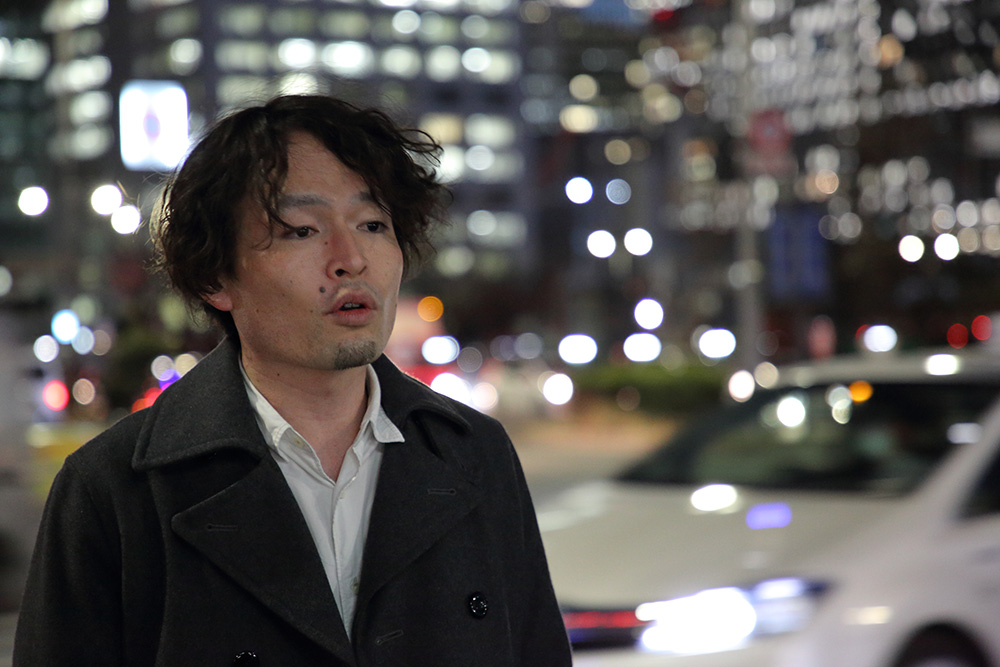
Category Theory for New QA Methods
The ERATO MMSD project couples logic with category theory to develop a method that manufacturers can use to assure the safety of their products.
“Category theory comes from algebra. It’s a mathematical language used for structural description,” said Prof. Hasuo, a category theory specialist. “It focuses on the relations among objects and expresses it in an abstract form. It differs from ‘graph theory,’ which also describes the relations among objects, in that category theory doesn’t generalize what’s present; it abstracts the logics behind what’s present. In other words, category theory is a meta language.”
Category theory is an integral part of mathematical structuralism. The theory matured at the same time as structural anthropology grew out of the study of algebraic structures in France during the 1940s. This historical fact may make one wonder if and how category theory and structural anthropology – which owes its development to Claude Lévi-Strauss – may be related to one another.
“Claude Lévi-Strauss (French anthropologist whose work helped the development of structuralism) made many contributions to the academic world, but I understand one of them was his idea that all kinds of communities – or mass bodies of things – have immutable structures supporting them. I believe the same is true for masses of data. Whether it’s a traditional information system – which is the focus of the data science – or an information system involving physics data of industrial machines, they both deal with collections of information. So, they’ve got to share some things in common from an algebraic perspective,” Prof. Hasuo said.
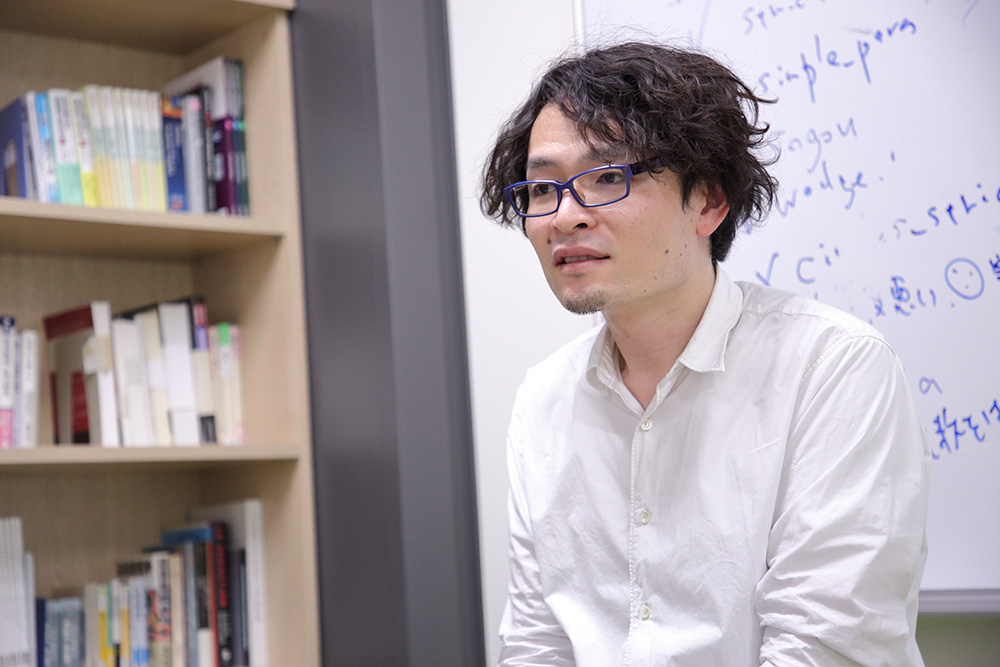
Mathematic Processes as Guide for Exploring Unfamiliar Territory
Prof. Hasuo’s is not the first researcher to suggest the use of formal methods as verification techniques for physical information systems. In fact, there have been many attempts made around the world to do just that. “Those attempts have not necessarily been unsuccessful because it is not clear how to make formal methods usable for cyber-physical information systems,” Prof. Hasuo said. He wants to use category theory to create the missing link, and that makes his idea and project exceptionally cutting-edge.
Formal methods – which are used to verify software does its job in the way it’s supposed to – are regarded as reliable quality-assurance techniques because they are mathematical, and therefore, leave little room for ambiguity and error. For manufacturers, minor inaccuracy could mean a disaster. Hair-splitting margins of error have indeed caused problems, such as rocket launching problems and arithmetic glitches in computers. Formal methods are useful for ensuring software products won’t get stuck in an infinite loop nor present any vulnerability. So, it could change the ways industries operate around the world if the ERATO MMSD project succeeds.
“Mathematical processes show you the ropes to guide you through the unknown territory,” Prof. Hasuo said. “We are going to use category theory to expand formal methods and create templates for defining problems in a mathematic language. We will gradually flesh them out and keep the templates in our ‘tool box,’ if you will, so we can take out suitable one, depending on what problem we need to solve, and work with manufacturing engineers to provide evidence for whatever they need to verify or prove. The ever-advancing AI and machine-learning technology can be useful for this process, as well,” he said. “When this is put to use, this will provide additional support for the current quality-assurance process centering around engineers’ judgment calls based on their years of experience and knowledge. This should drastically reduce the time required for verification processes.”
Prof. Hasuo and his team want to see five cases of manufacturing applications of this verification method before the ERATO MMSD project completes in 5 ½ years. The team is already discussing possible applications with several companies, according to Prof. Hasuo.
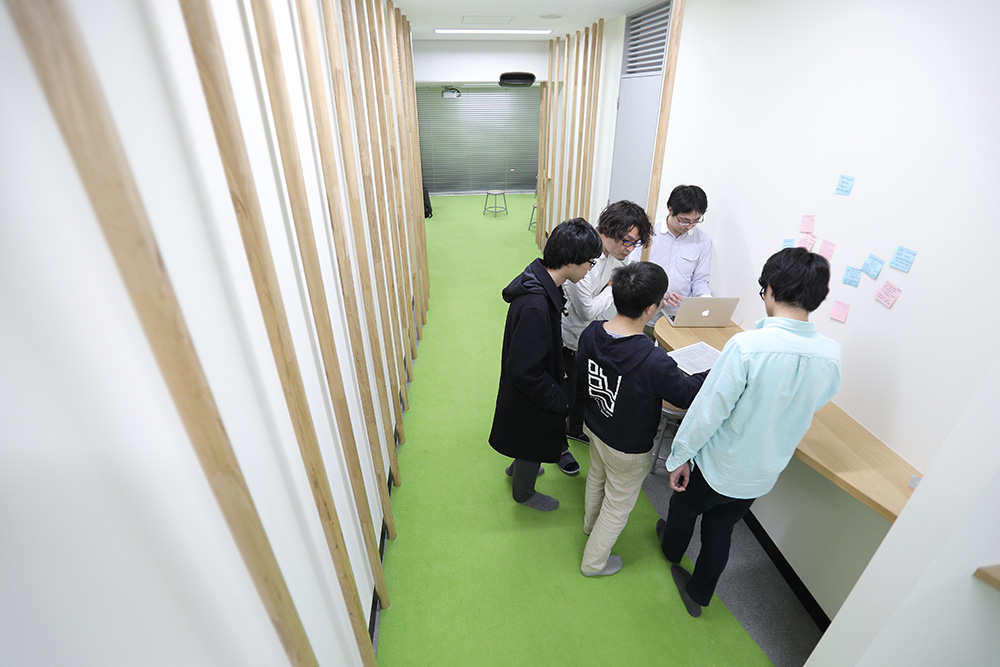
Interviewer: Rue Ikeya
Photographs: Yuji Iijima unless noted otherwise
Released on: August 10, 2018 (The Japanese version released on January 10, 2018)